- お役立ち記事
- Fundamentals of data-driven AI and application to image classification systems
Fundamentals of data-driven AI and application to image classification systems
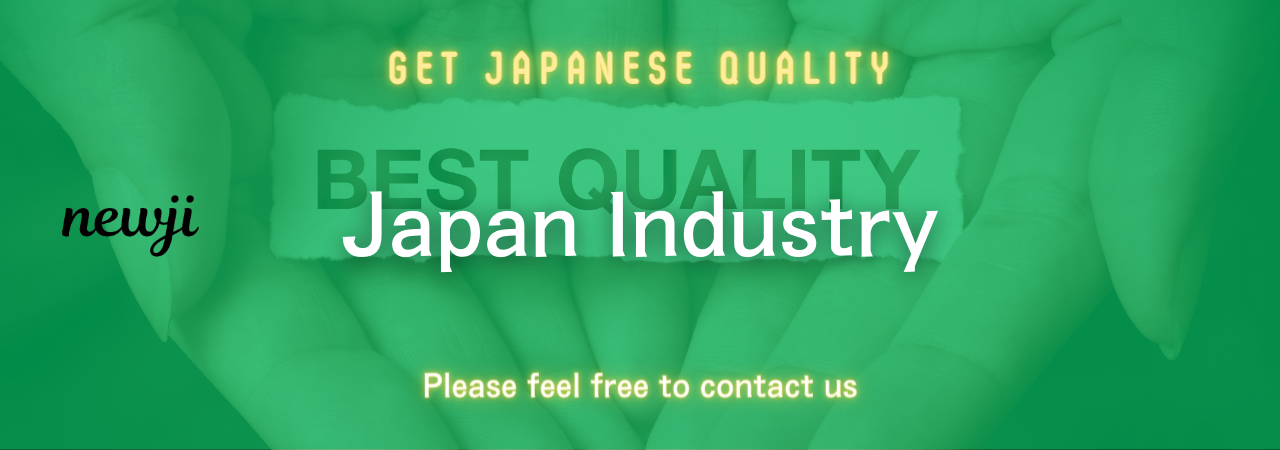
目次
Understanding Data-Driven AI
Data-driven AI refers to artificial intelligence systems that heavily rely on data to learn and make decisions.
These systems are designed to process large volumes of data to identify patterns and trends.
The data acts as the foundation that informs the algorithms, enabling them to perform specific tasks with accuracy.
Data-driven AI functions through a cycle of data collection, analysis, and application.
Initially, massive datasets are gathered, which serve as the primary input.
Data analysis then begins, where AI models comb through the data to detect essential features and hidden structures.
Finally, this information is applied, leading AI systems to make more informed decisions or predictions.
The Role of Data in AI
Data is the lifeblood of AI systems, providing the necessary fuel for machine learning algorithms.
The quality and quantity of data significantly impact the performance of AI models.
When AI is trained on a diverse and comprehensive dataset, it can offer more precise results.
Conversely, poor or insufficient data leads to biased or inaccurate outcomes.
A vital aspect of data-driven AI is the continuous influx of data.
As more data becomes available, AI systems update and improve over time.
This ensures that the solutions generated by the AI remain relevant and effective.
However, it’s also crucial to ensure the privacy and security of data, following ethical guidelines and laws.
AI’s Application in Image Classification Systems
Image classification is one of the critical applications of data-driven AI.
In image classification systems, AI utilizes image data to categorize objects within an image.
This technology is prevalent in various sectors, including healthcare, retail, and autonomous vehicles.
The process begins with the training of AI models using a substantial dataset of labeled images.
These labels clarify what each image represents, helping the AI to learn associations between visual patterns and their respective categories.
Once trained, the AI can identify and group new, unlabeled images based on its learned understanding.
For example, in a medical context, AI can classify medical images, differentiating between healthy cells and anomalies.
How AI Learns to Classify Images
The training process for an image classification system involves several steps:
– **Data Collection:** First, a large dataset of images, often labeled for specific categories, is gathered.
– **Preprocessing:** The collected images are then preprocessed to ensure consistency and quality.
This might involve resizing, normalizing, or augmenting images.
– **Training the Model:** A neural network, usually a convolutional neural network (CNN), is employed to learn from the training data.
During training, the network adjusts its weights using techniques such as backpropagation to minimize errors.
– **Testing and Validation:** Once the model is trained, it is tested with new image data to evaluate its accuracy and performance.
– **Deployment:** After thorough testing, the AI model is deployed for practical use, where it can classify images in real-time or upon request.
Challenges in Developing Image Classification Systems
While image classification AI systems can be highly effective, several challenges can arise:
– **Data Diversity:** Ensuring that the dataset represents a wide range of scenarios is crucial.
Without diversity, AI can develop biases, leading to inaccuracies.
– **Computational Resources:** Training complex models requires significant computational power and time.
Access to high-performance computing resources is often necessary.
– **Interpretability:** AI systems can be like black boxes, making it difficult to understand the reasoning behind specific classifications.
This lack of transparency can be problematic, particularly in sensitive sectors like healthcare.
– **Continuous Learning:** In dynamic fields, the AI system must adapt to new data and evolving categories.
The Future of AI in Image Classification
As technology advances, the application of AI in image classification will become even more sophisticated.
Future developments could involve enhanced algorithms that require less computational power and deliver faster results.
Additionally, there could be increased focus on developing methods to interpret and explain AI decisions, improving trust and transparency.
Innovations may also lead to AI systems that can autonomously update with new categories, maintaining relevance without manual intervention.
Moreover, advancements in quantum computing could radically reduce the processing time and resources needed for training AI models.
In conclusion, understanding the fundamentals of data-driven AI and its application in image classification systems highlights its transformative potential.
While there are challenges to overcome, the future promises exciting developments that can reshape industries and enhance everyday life.
Continued research and innovation will be essential in harnessing the full capabilities of AI technology.
資料ダウンロード
QCD調達購買管理クラウド「newji」は、調達購買部門で必要なQCD管理全てを備えた、現場特化型兼クラウド型の今世紀最高の購買管理システムとなります。
ユーザー登録
調達購買業務の効率化だけでなく、システムを導入することで、コスト削減や製品・資材のステータス可視化のほか、属人化していた購買情報の共有化による内部不正防止や統制にも役立ちます。
NEWJI DX
製造業に特化したデジタルトランスフォーメーション(DX)の実現を目指す請負開発型のコンサルティングサービスです。AI、iPaaS、および先端の技術を駆使して、製造プロセスの効率化、業務効率化、チームワーク強化、コスト削減、品質向上を実現します。このサービスは、製造業の課題を深く理解し、それに対する最適なデジタルソリューションを提供することで、企業が持続的な成長とイノベーションを達成できるようサポートします。
オンライン講座
製造業、主に購買・調達部門にお勤めの方々に向けた情報を配信しております。
新任の方やベテランの方、管理職を対象とした幅広いコンテンツをご用意しております。
お問い合わせ
コストダウンが利益に直結する術だと理解していても、なかなか前に進めることができない状況。そんな時は、newjiのコストダウン自動化機能で大きく利益貢献しよう!
(Β版非公開)