- お役立ち記事
- Deep learning technology for detection, identification, and classification of facial and human images
Deep learning technology for detection, identification, and classification of facial and human images
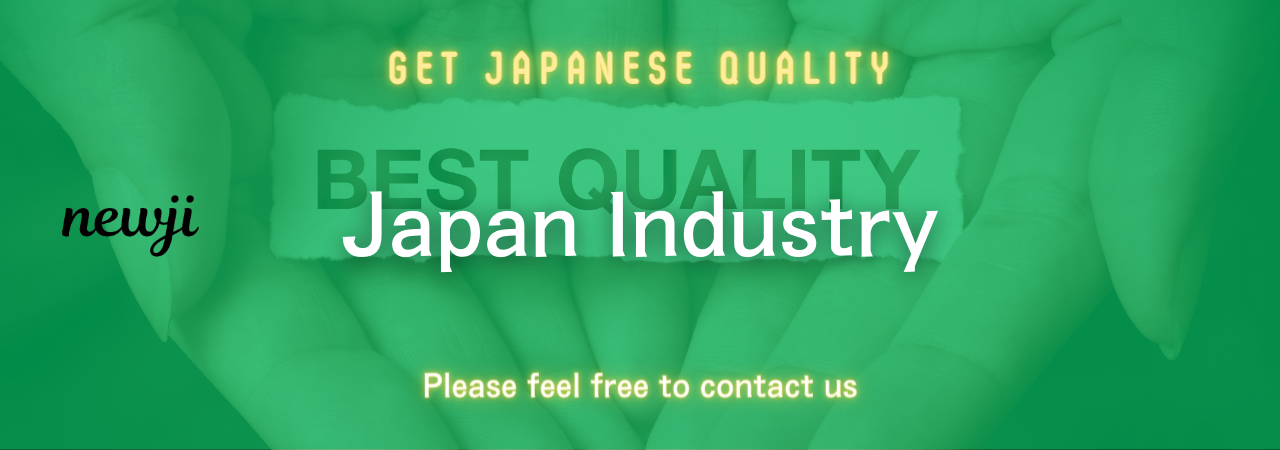
目次
Understanding Deep Learning Technology
Deep learning is a powerful subset of machine learning that is inspired by the structure and function of the human brain.
It involves neural networks with three or more layers which allow it to process and analyze data with higher levels of accuracy.
In recent years, deep learning technology has significantly evolved, especially in the field of image detection, identification, and classification, particularly when it comes to facial and human images.
The Basics of Deep Learning in Image Processing
At its core, deep learning utilizes artificial neural networks to learn and predict outcomes.
For image processing, these neural networks analyze vast amounts of labelled data to understand patterns, shapes, and features within images.
This data is used to teach networks how to differentiate between different objects within images, like understanding the nuances of a person’s face or the shape of a human figure.
A key feature of deep learning is its ability to improve its performance as it is exposed to more data.
This makes it particularly useful for image processing where there is a high demand for precision.
Facial Recognition Using Deep Learning
Facial recognition is one of the most common applications of deep learning technology.
It involves identifying and verifying people based on their facial features.
Deep learning significantly enhances the accuracy of facial recognition systems by automatically extracting features from images and improving understanding through continuous learning.
In practical applications, deep learning-based facial recognition systems are used in security systems, social media platforms, and even smartphones for user authentication.
These systems focus on key facial landmarks, such as the distance between the eyes, the width of the nose, and the shape of the cheekbones.
Algorithms can then recognize and verify an individual’s identity by comparing these features to stored data.
Human Image Classification with Deep Learning
The classification of human images goes beyond just recognizing faces.
It involves categorizing images based on different human attributes, such as gender, age, and emotions, as well as identifying human activities like running, jumping, or sitting.
Deep learning models are trained on large datasets that include varied images of humans to accurately classify and predict such attributes.
For example, convolutional neural networks (CNNs) are extensively used for image classification tasks.
They are effective in automatically detecting features in images without any manual intervention, making the process more efficient and accurate.
Challenges in Deep Learning for Image Detection
While deep learning offers significant advancements in image detection and classification, it also poses some challenges.
First, deep learning models require vast amounts of data for training, which can be hard to collect and manage.
High-quality images with properly annotated labels are essential to training effective models.
Another challenge is computational power.
Deep learning algorithms are resource-intensive and require powerful hardware to process large datasets quickly.
This necessitates the use of specialized software and equipment, which can be expensive and limiting for some applications.
Additionally, there are concerns about privacy and security when it comes to facial recognition technology.
Ensuring that data is used ethically and appropriately is crucial, and there are ongoing debates about the balance between technological advancement and individual privacy rights.
Advancements and Future Prospects
Despite these challenges, advancements in deep learning continue at a rapid pace.
Researchers are actively working on various approaches to make deep learning more robust, interpretable, and less data-dependent.
Techniques such as transfer learning, which allows models to use pre-existing knowledge to solve new but related tasks, are being adopted to minimize the need for large datasets.
Moreover, real-time processing of images using deep learning is becoming more feasible, opening up new possibilities for applications like autonomous vehicles, augmented reality, and healthcare diagnostics.
The potential for deep learning in sectors like retail, marketing, and entertainment is vast, as businesses seek to leverage the power of image and facial recognition to enhance customer experiences.
Conclusion
Deep learning technology is at the forefront of innovation in image detection, identification, and classification.
It has made significant contributions to improving the accuracy and efficiency of facial and human recognition systems.
While challenges like data requirements and privacy concerns remain, ongoing research and advancements promise exciting developments in the field.
As technology continues to advance, we can expect deep learning to play an integral role in the evolving landscape of artificial intelligence and machine learning, offering new opportunities and solutions across various industries.
資料ダウンロード
QCD調達購買管理クラウド「newji」は、調達購買部門で必要なQCD管理全てを備えた、現場特化型兼クラウド型の今世紀最高の購買管理システムとなります。
ユーザー登録
調達購買業務の効率化だけでなく、システムを導入することで、コスト削減や製品・資材のステータス可視化のほか、属人化していた購買情報の共有化による内部不正防止や統制にも役立ちます。
NEWJI DX
製造業に特化したデジタルトランスフォーメーション(DX)の実現を目指す請負開発型のコンサルティングサービスです。AI、iPaaS、および先端の技術を駆使して、製造プロセスの効率化、業務効率化、チームワーク強化、コスト削減、品質向上を実現します。このサービスは、製造業の課題を深く理解し、それに対する最適なデジタルソリューションを提供することで、企業が持続的な成長とイノベーションを達成できるようサポートします。
オンライン講座
製造業、主に購買・調達部門にお勤めの方々に向けた情報を配信しております。
新任の方やベテランの方、管理職を対象とした幅広いコンテンツをご用意しております。
お問い合わせ
コストダウンが利益に直結する術だと理解していても、なかなか前に進めることができない状況。そんな時は、newjiのコストダウン自動化機能で大きく利益貢献しよう!
(Β版非公開)