- お役立ち記事
- Modeling with state space models
Modeling with state space models
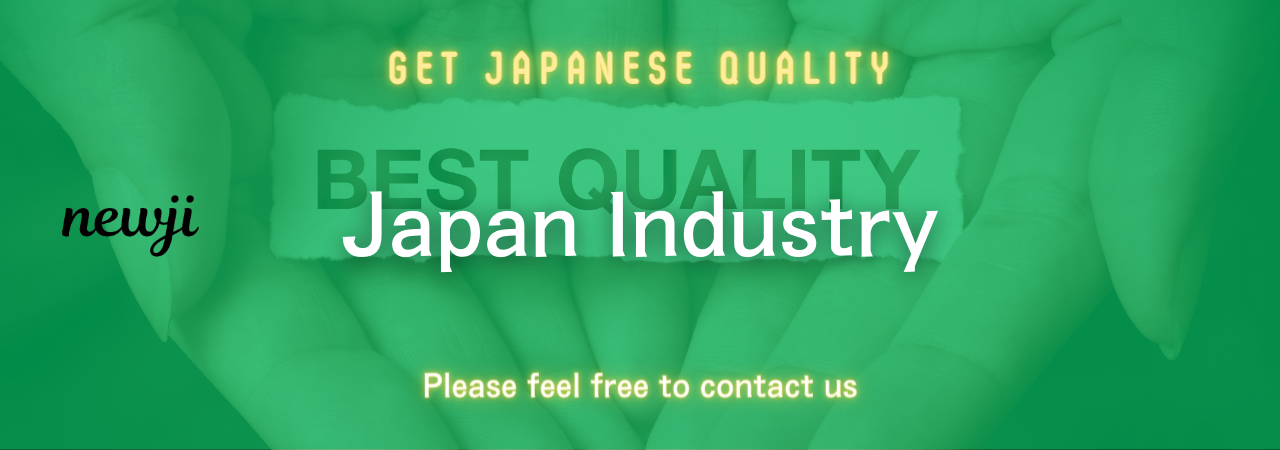
目次
What Are State Space Models?
State space models are powerful tools used in various fields, including engineering, economics, and statistics, to model dynamic systems.
These models are used to describe how a system changes over time.
The key idea behind state space models is to represent a system by a set of equations that describe the evolution of “state variables.”
These state variables capture all the relevant information needed to describe the system’s future behavior.
In simple terms, state space models help us understand how things change and predict future behavior based on past observations.
They are widely used in forecasting, control systems, and time series analysis.
Components of State Space Models
State space models comprise two main equations: the state equation and the observation equation.
State Equation
The state equation describes how the state variables evolve over time.
It is a mathematical representation of the system dynamics.
The state equation is typically expressed as:
x(t) = A * x(t-1) + B * u(t-1) + w(t)
Here, x(t) is the current state vector, A is the state transition matrix, u(t-1) represents input variables or control signals, and w(t) is the process noise that captures uncertainties in the system.
Observation Equation
The observation equation links the observable data to the hidden state variables.
It is often represented as:
y(t) = C * x(t) + v(t)
In this equation, y(t) is the observed data, C is the observation matrix, and v(t) is the observation noise, accounting for measurement errors or external influences.
Applications of State Space Models
State space models have a wide range of applications, making them indispensable in various domains.
Control Systems
In control systems engineering, state space models are extensively used to design and analyze control strategies for dynamic systems.
Engineers apply these models to achieve desired system behavior by adjusting input signals based on feedback from system states.
Econometrics
Economists utilize state space models to analyze economic time series data.
These models help in understanding trends, cycles, and other dynamic behaviors in economic data, allowing for better forecasting and decision-making.
Signal Processing
In signal processing, state space models are used to filter and smooth data.
They play a critical role in noise reduction and enhancing signal quality in various applications, such as audio and image processing.
Robotics and Navigation
Robotic systems and autonomous vehicles often rely on state space models for navigation and control.
These models help robots and vehicles make real-time decisions, ensuring accurate and efficient movement in dynamic environments.
Advantages of State Space Models
State space models offer several advantages, which contribute to their popularity among researchers and practitioners.
Flexibility
One of the key advantages of state space models is their flexibility.
They can accommodate nonlinear relationships and handle multiple input variables, making them suitable for complex systems.
Noise Handling
State space models efficiently handle noise in both the process and observation stages.
By incorporating noise terms in the equations, these models provide a robust framework for dealing with uncertainties.
Predictive Power
State space models excel in predicting future states and outputs.
Their use of state variables provides a deeper understanding of system dynamics, resulting in accurate forecasts.
Challenges in Using State Space Models
While state space models are powerful, they also present certain challenges that need careful consideration.
Complexity
The mathematical complexity of state space models can be intimidating, especially for beginners.
Understanding the matrix operations and system equations requires a solid grasp of linear algebra and mathematical concepts.
Parameter Estimation
Estimating the parameters of state space models requires accurate data and suitable estimation techniques.
The process can be computationally intensive, affecting model accuracy if not done correctly.
Model Selection
Choosing the right model structure is crucial for accurate predictions.
Selecting an inappropriate model can lead to poor performance and unreliable results.
How to Build a State Space Model
Building a state space model involves several steps, which are crucial for a successful modeling process.
Define the System
The first step is to clearly define the system you are interested in modeling.
Identify the state variables, inputs, and outputs based on the system’s purpose and dynamics.
Formulate the Equations
Develop the state and observation equations by understanding the relationships among variables.
This involves determining the state transition matrix, observation matrix, and noise terms.
Collect Data
Gather relevant data for your system, which will be used to estimate the model parameters.
Ensure the data is comprehensive and accurate to improve model performance.
Estimate Parameters
Apply suitable techniques to estimate the parameters of your state space model.
Common methods include Kalman filters and maximum likelihood estimation, depending on the model complexity and data availability.
Validate the Model
It’s essential to validate the model by comparing its predictions with observed data.
Check the model’s performance and make adjustments if necessary to improve accuracy.
Conclusion
State space models offer a versatile framework for modeling dynamic systems.
Their ability to handle noise and provide accurate predictions makes them valuable in various domains, from control systems to econometrics.
By understanding the components and challenges of state space models, you can harness their power to gain insights into complex systems and make informed decisions.
Whether you’re an engineer, economist, or data scientist, state space models are tools worth mastering to analyze and predict the behavior of dynamic systems.
資料ダウンロード
QCD調達購買管理クラウド「newji」は、調達購買部門で必要なQCD管理全てを備えた、現場特化型兼クラウド型の今世紀最高の購買管理システムとなります。
ユーザー登録
調達購買業務の効率化だけでなく、システムを導入することで、コスト削減や製品・資材のステータス可視化のほか、属人化していた購買情報の共有化による内部不正防止や統制にも役立ちます。
NEWJI DX
製造業に特化したデジタルトランスフォーメーション(DX)の実現を目指す請負開発型のコンサルティングサービスです。AI、iPaaS、および先端の技術を駆使して、製造プロセスの効率化、業務効率化、チームワーク強化、コスト削減、品質向上を実現します。このサービスは、製造業の課題を深く理解し、それに対する最適なデジタルソリューションを提供することで、企業が持続的な成長とイノベーションを達成できるようサポートします。
オンライン講座
製造業、主に購買・調達部門にお勤めの方々に向けた情報を配信しております。
新任の方やベテランの方、管理職を対象とした幅広いコンテンツをご用意しております。
お問い合わせ
コストダウンが利益に直結する術だと理解していても、なかなか前に進めることができない状況。そんな時は、newjiのコストダウン自動化機能で大きく利益貢献しよう!
(Β版非公開)