- お役立ち記事
- Materials informatics and application points from small-scale experimental data
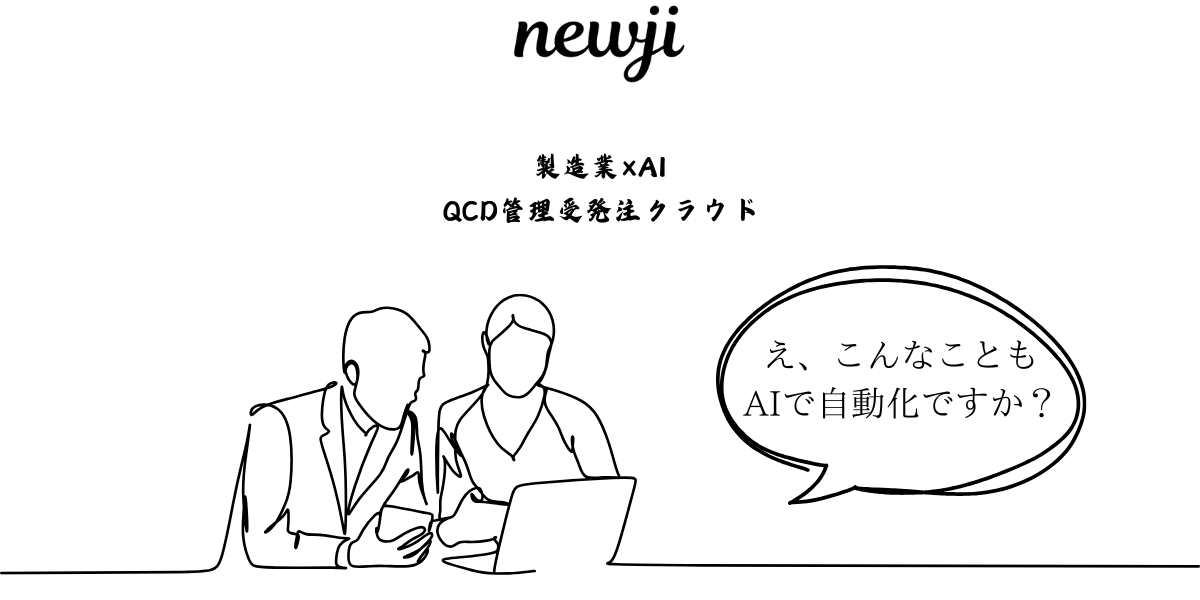
Materials informatics and application points from small-scale experimental data
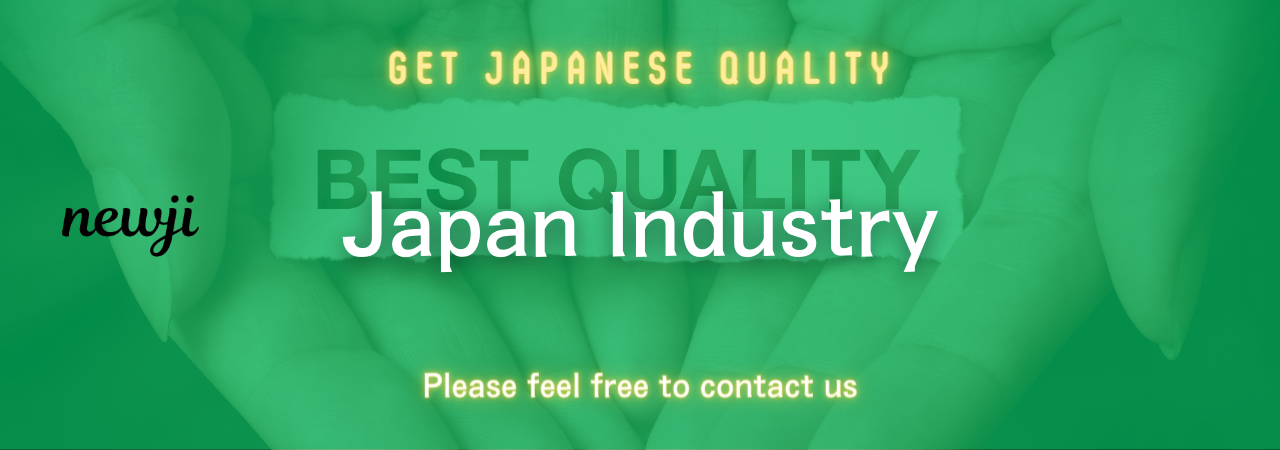
目次
Understanding Materials Informatics
Materials informatics is an emerging field that combines material science with data science techniques to understand and predict material properties and behaviors.
This interdisciplinary approach leverages algorithms, machine learning, and other computational tools to analyze and model data.
The goal is to accelerate the discovery and development of new materials by providing insights that would be difficult or time-consuming to obtain through traditional experimental methods.
The Role of Small-Scale Experimental Data
Small-scale experimental data plays a crucial role in the development of materials informatics.
While large datasets are often ideal for training machine learning models, small-scale data can also provide significant insights, especially when curated and analyzed properly.
In many cases, small-scale data may be the only available source, particularly in specialized or novel research areas.
This data can be crucial in developing initial models that can be refined as more information becomes available.
Getting Started with Small-Scale Data
When working with small-scale experimental data, the first step is to ensure data quality and consistency.
This involves careful data collection and cleaning to minimize errors and inconsistencies.
Another important aspect is data augmentation.
This technique involves generating additional data points based on existing data through methods such as interpolation, small transformations, or simulations.
These augmented datasets can help build more robust models and improve prediction accuracy.
Leveraging Machine Learning in Materials Informatics
Machine learning is at the heart of materials informatics, providing tools for uncovering patterns and relationships within data.
With small-scale data, specific strategies must be employed to effectively utilize machine learning.
One common approach is transfer learning, where models trained on large datasets in related fields are adapted to work with smaller datasets.
This strategy helps in harnessing the power of pre-trained models, saving time and resources.
Another approach is the use of Bayesian models, which can handle uncertainty and make reliable predictions even with limited data.
These models are particularly useful in materials science where experimental conditions can vary widely.
Practical Applications of Materials Informatics
Materials informatics is being applied in numerous fields, from electronics and pharmaceuticals to automotive and aerospace industries.
One key application is the accelerated discovery of novel materials.
By predicting material properties and behaviors faster than traditional methods, researchers can develop new compounds and composites with desired properties.
In electronics, materials informatics aids in the development of semiconductors by predicting their performance and stability under different conditions.
Similarly, in the pharmaceutical industry, it helps in the identification of new drug formulations by modeling interactions at the molecular level.
In the automotive and aerospace industries, materials informatics supports the design of lightweight yet strong materials, leading to more efficient and sustainable transportation solutions.
Challenges and Future Directions
Despite its potential, materials informatics faces several challenges.
A major challenge is the integration of diverse data types ranging from numerical and image data to textual and historical records.
Creating universal standards for data format and storage can facilitate better data sharing and collaboration across the field.
Another challenge is the interpretability of machine learning models.
While these models can predict material properties, understanding the reasoning behind their predictions is essential for gaining scientific insights and ensuring trust among researchers.
Looking forward, the field will benefit from advancements in machine learning algorithms, improved computational resources, and increased collaboration among researchers from various disciplines.
As more data becomes available and methods improve, materials informatics will play an increasingly important role in driving innovation and sustainability in material science.
Conclusion
Materials informatics offers exciting possibilities for revolutionizing how we approach material discovery and development.
Through the effective use of small-scale experimental data and advanced computational techniques, the process of identifying and optimizing new materials can be significantly expedited.
Despite challenges, the collaboration of data scientists and material scientists promises a future where material discoveries are not limited by the speed and scale of data collection.
As we continue to unlock the potential of materials informatics, industries across the globe can expect to see substantial advancements in technology and sustainability.
資料ダウンロード
QCD調達購買管理クラウド「newji」は、調達購買部門で必要なQCD管理全てを備えた、現場特化型兼クラウド型の今世紀最高の購買管理システムとなります。
ユーザー登録
調達購買業務の効率化だけでなく、システムを導入することで、コスト削減や製品・資材のステータス可視化のほか、属人化していた購買情報の共有化による内部不正防止や統制にも役立ちます。
NEWJI DX
製造業に特化したデジタルトランスフォーメーション(DX)の実現を目指す請負開発型のコンサルティングサービスです。AI、iPaaS、および先端の技術を駆使して、製造プロセスの効率化、業務効率化、チームワーク強化、コスト削減、品質向上を実現します。このサービスは、製造業の課題を深く理解し、それに対する最適なデジタルソリューションを提供することで、企業が持続的な成長とイノベーションを達成できるようサポートします。
オンライン講座
製造業、主に購買・調達部門にお勤めの方々に向けた情報を配信しております。
新任の方やベテランの方、管理職を対象とした幅広いコンテンツをご用意しております。
お問い合わせ
コストダウンが利益に直結する術だと理解していても、なかなか前に進めることができない状況。そんな時は、newjiのコストダウン自動化機能で大きく利益貢献しよう!
(Β版非公開)