- お役立ち記事
- 2D/3D SLAM algorithms and implementation
2D/3D SLAM algorithms and implementation
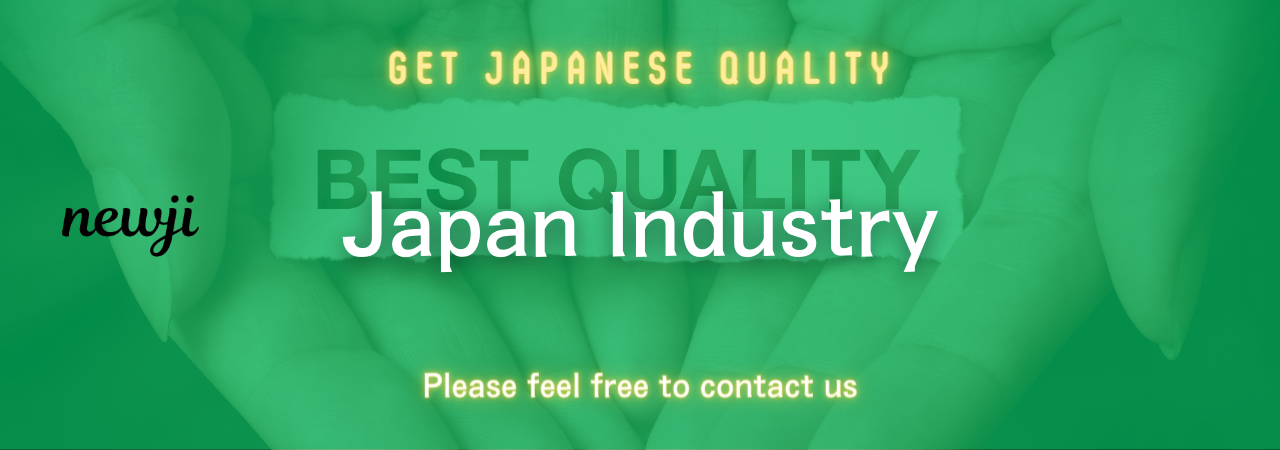
目次
Understanding SLAM Algorithms
SLAM, which stands for Simultaneous Localization and Mapping, is a critical component in robotics and autonomous systems.
It enables a robot or device to map an unknown environment while keeping track of its location within that space simultaneously.
This process is essential for robots to navigate through environments autonomously and perform tasks without human intervention.
There are two primary types of SLAM algorithms: 2D SLAM and 3D SLAM.
The main difference between these lies in the dimensions of the environment they map.
2D SLAM is typically used when the environment is relatively flat, such as indoor navigation on a single floor.
On the other hand, 3D SLAM is employed for more complex environments where the robot needs to perceive depth or traverse through different elevation levels, like in multi-story buildings or outdoor environments with uneven terrain.
2D SLAM Algorithms
2D SLAM algorithms are generally more straightforward compared to their 3D counterparts.
These algorithms rely on two-dimensional sensor data obtained from lasers or cameras, which help generate a map and estimate the robot’s position within that map.
There are a few commonly used 2D SLAM algorithms, each with its strengths and optimal use cases.
EKF-SLAM
Extended Kalman Filter SLAM (EKF-SLAM) is one of the most traditional methods used in 2D SLAM.
This algorithm uses probabilistic models to estimate the robot’s position and the environmental map.
The EKF-SLAM is advantageous because it handles noisy sensor data effectively.
However, it suffers from computational limitations when scaling to larger environments due to its increasing complexity.
FastSLAM
FastSLAM is an extension of the particle filter approach that improves over the EKF-SLAM by maintaining multiple hypotheses of the robot’s path.
It uses a particle filter to represent the robot’s path and a set of Kalman filters for estimating the locations of landmarks.
FastSLAM excels in environments with a significant number of landmarks and is computationally more efficient than EKF-SLAM.
However, it requires managing a large number of particles, which can become challenging in resource-constrained devices.
GMapping
GMapping is a widely-used 2D SLAM algorithm known for its efficiency and accuracy in indoor environments.
It makes use of laser range-finders to perform grid-based mapping.
GMapping excels in generating detailed maps and is particularly popular in robot platforms that need reliable indoor navigation.
Nevertheless, it can struggle in feature-sparse environments and requires a good initial guess for better performance.
3D SLAM Algorithms
3D SLAM algorithms add an extra dimension to the mapping process, enabling robots to navigate environments with varying elevations and complex structures.
Handling 3D data requires more sophisticated mathematical models and advanced computation techniques, making 3D SLAM inherently more complex than 2D.
RGB-D SLAM
RGB-D SLAM combines color (RGB) and depth (D) data to create a dense and textured 3D map.
This method typically employs sensors like Microsoft’s Kinect, which captures both visual and depth information.
The main advantage of RGB-D SLAM is its ability to create visually rich 3D maps, but it can be susceptible to errors in environments with poor lighting or reflective surfaces.
LiDAR SLAM
LiDAR SLAM uses LiDAR sensors to measure distances with laser beams and create precise 3D point clouds of the environment.
LiDAR SLAM algorithms like Hector SLAM or Cartographer are known for their high accuracy and reliability in outdoor environments.
Although LiDAR sensors provide excellent range and accuracy, they are generally more expensive than other sensors, making them less feasible for cost-sensitive applications.
ORB-SLAM2
ORB-SLAM2 is a versatile visual SLAM algorithm that performs well in 3D environments using only a single camera without relying on other sensor inputs.
It leverages keypoints and descriptors to track the environment and perceives depth through visual cues.
ORB-SLAM2 is highly adaptable for different scenarios but can struggle in scenarios with repetitive patterns or low-texture surfaces.
Implementing SLAM Algorithms
Implementing SLAM algorithms requires a rigorous understanding of both the theoretical aspects and practical considerations of robotics.
Successful implementation depends on several factors such as the choice of sensors, computational resources, and the intended environment.
Choosing the Right Sensors
The type of sensors used plays a significant role in the performance of the SLAM system.
For 2D SLAM, laser range finders or cameras may suffice, while 3D SLAM systems might require additional depth sensors like LiDAR or RGB-D cameras.
Choosing the right sensors involves considering factors like the environment, range, accuracy, and budget.
Processing Power and Resources
SLAM algorithms can be computationally intensive, especially when scaling to larger environments and adding more dimensions.
The choice of processing platform, be it embedded systems or high-performance CPUs/GPUs, is crucial in ensuring real-time performance and efficiency.
Likewise, optimizing the algorithm to leverage available resources effectively is essential for smooth operation.
Testing and Calibration
Thorough testing and calibration are critical for ensuring the reliability and accuracy of a SLAM system.
Calibration of sensors ensures precise data is inputted into the algorithm, while extensive field testing helps fine-tune performance based on real-world scenarios.
Simulations and controlled environment testing are also useful for debugging and improving the algorithm’s robustness.
Conclusion
SLAM algorithms have opened a new era of possibilities for autonomous robotics and navigation systems.
Choosing between 2D and 3D SLAM depends greatly on the application and environment, with each offering specific advantages.
Understanding the key differences, implementation challenges, and sensor capabilities allows developers to create reliable and efficient SLAM systems suited for a wide range of applications.
As technology continues to evolve, the future of SLAM promises even more innovation and capability in autonomous systems.
資料ダウンロード
QCD調達購買管理クラウド「newji」は、調達購買部門で必要なQCD管理全てを備えた、現場特化型兼クラウド型の今世紀最高の購買管理システムとなります。
ユーザー登録
調達購買業務の効率化だけでなく、システムを導入することで、コスト削減や製品・資材のステータス可視化のほか、属人化していた購買情報の共有化による内部不正防止や統制にも役立ちます。
NEWJI DX
製造業に特化したデジタルトランスフォーメーション(DX)の実現を目指す請負開発型のコンサルティングサービスです。AI、iPaaS、および先端の技術を駆使して、製造プロセスの効率化、業務効率化、チームワーク強化、コスト削減、品質向上を実現します。このサービスは、製造業の課題を深く理解し、それに対する最適なデジタルソリューションを提供することで、企業が持続的な成長とイノベーションを達成できるようサポートします。
オンライン講座
製造業、主に購買・調達部門にお勤めの方々に向けた情報を配信しております。
新任の方やベテランの方、管理職を対象とした幅広いコンテンツをご用意しております。
お問い合わせ
コストダウンが利益に直結する術だと理解していても、なかなか前に進めることができない状況。そんな時は、newjiのコストダウン自動化機能で大きく利益貢献しよう!
(Β版非公開)