- お役立ち記事
- Fundamentals of Bayesian statistics and subjective probability
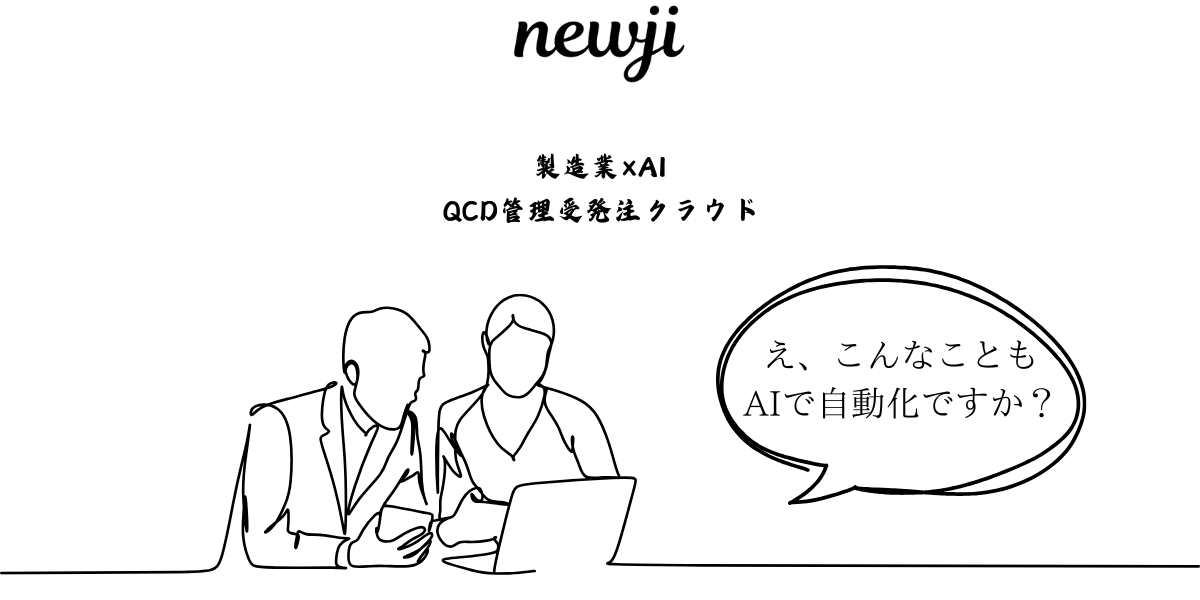
Fundamentals of Bayesian statistics and subjective probability
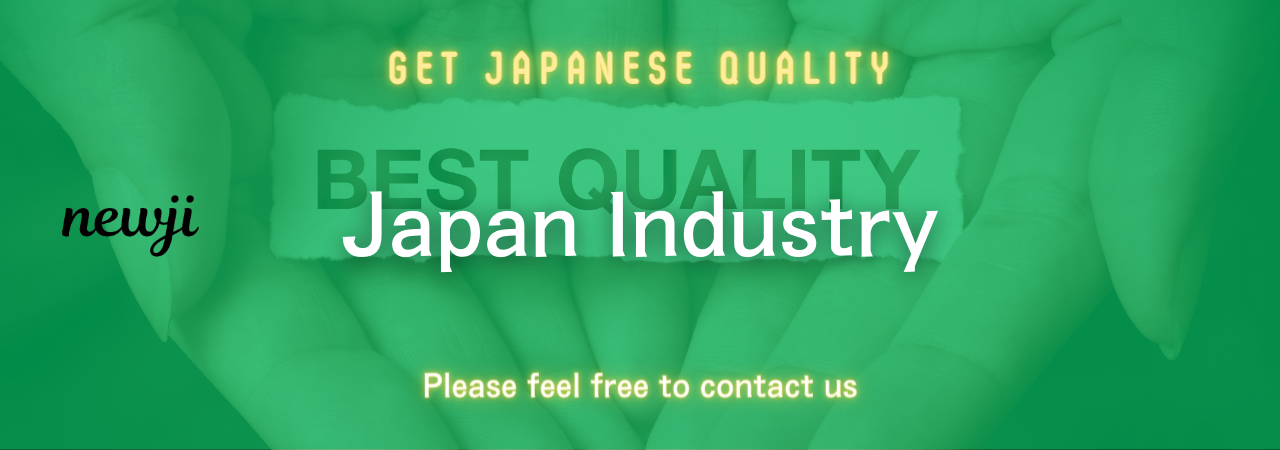
目次
Understanding Bayesian Statistics
Bayesian statistics is a fascinating area of mathematics that deals with probabilities.
It’s named after the Reverend Thomas Bayes, who formulated a specific theorem that provides a way to update the probability of a hypothesis based on new evidence.
Unlike classical or frequentist statistics, which treats probability strictly as a frequency of occurrence, Bayesian statistics introduces a more flexible concept.
It allows for the incorporation of prior knowledge or beliefs into the probability analysis.
The Core of Bayesian Statistics
At the heart of Bayesian statistics lies Bayes’ Theorem.
This theorem essentially describes how to update the probability of a hypothesis as more evidence or information becomes available.
It is mathematically expressed as:
\[ P(H|E) = \frac{P(E|H) \cdot P(H)}{P(E)} \]
Here, \( P(H|E) \) is the posterior probability, or the probability of the hypothesis \( H \) given the evidence \( E \).
\( P(E|H) \) is the likelihood, or the probability of observing the evidence \( E \) under the hypothesis \( H \).
\( P(H) \) is the prior probability, which represents our initial belief about the hypothesis before seeing the evidence.
Finally, \( P(E) \) is the marginal likelihood, ensuring the probabilities sum to 1.
Subjective Probability Explained
Subjective probability provides a personal view on how likely an event is to occur.
Unlike objective probability, which is based entirely on previously observed frequencies or logical analysis, subjective probability is influenced by personal judgment or opinion.
For example, if you believe there’s a 70% chance it will rain tomorrow based on your interpretation of the weather forecast, that’s a subjective probability.
Integration with Bayesian Statistics
Bayesian statistics leverages subjective probabilities effectively.
The prior probability in Bayes’ Theorem is inherently subjective.
It reflects our beliefs or knowledge about a situation before we encounter new data.
As we gather new information, Bayes’ Theorem guides how we should adjust these beliefs in a systematic way.
An advantage of this framework is its ability to handle situations where data is sparse or unavailable.
For instance, in fields such as medicine or economic forecasting, Bayesian methods can incorporate expert opinions as prior probabilities to make informed predictions.
Applications of Bayesian Statistics
Bayesian statistics is widely used in various fields, from scientific research to machine learning.
Scientific Research
In scientific research, Bayesian methods provide a powerful tool for hypothesis testing and model comparison.
Scientists often face situations where data is incomplete or noisy.
Bayesian models help account for these uncertainties by integrating expert knowledge and new evidence.
Machine Learning
In machine learning, Bayesian approaches are used for developing algorithms that improve continuously.
These algorithms, such as Bayesian networks and Gaussian processes, help in predicting outcomes and making decisions under uncertainty.
For instance, spam filters often employ Bayesian techniques to update their models as they process more emails.
Risk Management
Risk management also benefits from Bayesian statistics.
Companies can leverage Bayesian models to forecast risks and make informed decisions.
By continuously updating risk evaluations as new data becomes available, organizations can better prepare for potential challenges.
Advantages of Bayesian Statistics
Bayesian statistics offers several advantages over traditional methods.
Incorporation of Prior Knowledge
One of the most significant advantages is the ability to incorporate prior knowledge.
This is particularly useful in fields where prior data or expert judgment can provide valuable context.
Flexibility in Handling Complex Problems
Bayesian approaches are highly flexible and can be tailored to a wide range of complex problems, such as those involving multiple variables or intricate relationships.
Adaptability to New Data
Bayesian models are inherently adaptive.
They can be updated easily with new data, which makes them particularly well-suited for dynamic environments where conditions change over time.
Challenges and Considerations
Despite their power, Bayesian methods come with certain challenges.
Computational Complexity
One of the main challenges is the computational complexity involved in solving Bayesian models, especially as datasets grow larger.
Advanced techniques and powerful computational resources are often required.
Subjectivity in Prior Distributions
Another consideration is the subjectivity introduced through prior distributions.
Different people might have different priors based on their personal beliefs, which can lead to variation in outcomes.
Therefore, it’s crucial to carefully justify and document the choice of priors.
In conclusion, Bayesian statistics offers a robust framework for dealing with uncertainty and integrating new information with existing knowledge.
Its ability to incorporate subjective probability provides flexibility and adaptability across various applications.
As computational tools evolve, the adoption of Bayesian methods is likely to continue expanding, offering deeper insights and more accurate predictions.
資料ダウンロード
QCD調達購買管理クラウド「newji」は、調達購買部門で必要なQCD管理全てを備えた、現場特化型兼クラウド型の今世紀最高の購買管理システムとなります。
ユーザー登録
調達購買業務の効率化だけでなく、システムを導入することで、コスト削減や製品・資材のステータス可視化のほか、属人化していた購買情報の共有化による内部不正防止や統制にも役立ちます。
NEWJI DX
製造業に特化したデジタルトランスフォーメーション(DX)の実現を目指す請負開発型のコンサルティングサービスです。AI、iPaaS、および先端の技術を駆使して、製造プロセスの効率化、業務効率化、チームワーク強化、コスト削減、品質向上を実現します。このサービスは、製造業の課題を深く理解し、それに対する最適なデジタルソリューションを提供することで、企業が持続的な成長とイノベーションを達成できるようサポートします。
オンライン講座
製造業、主に購買・調達部門にお勤めの方々に向けた情報を配信しております。
新任の方やベテランの方、管理職を対象とした幅広いコンテンツをご用意しております。
お問い合わせ
コストダウンが利益に直結する術だと理解していても、なかなか前に進めることができない状況。そんな時は、newjiのコストダウン自動化機能で大きく利益貢献しよう!
(Β版非公開)