- お役立ち記事
- Markov chain Monte Carlo method (MCMC method)
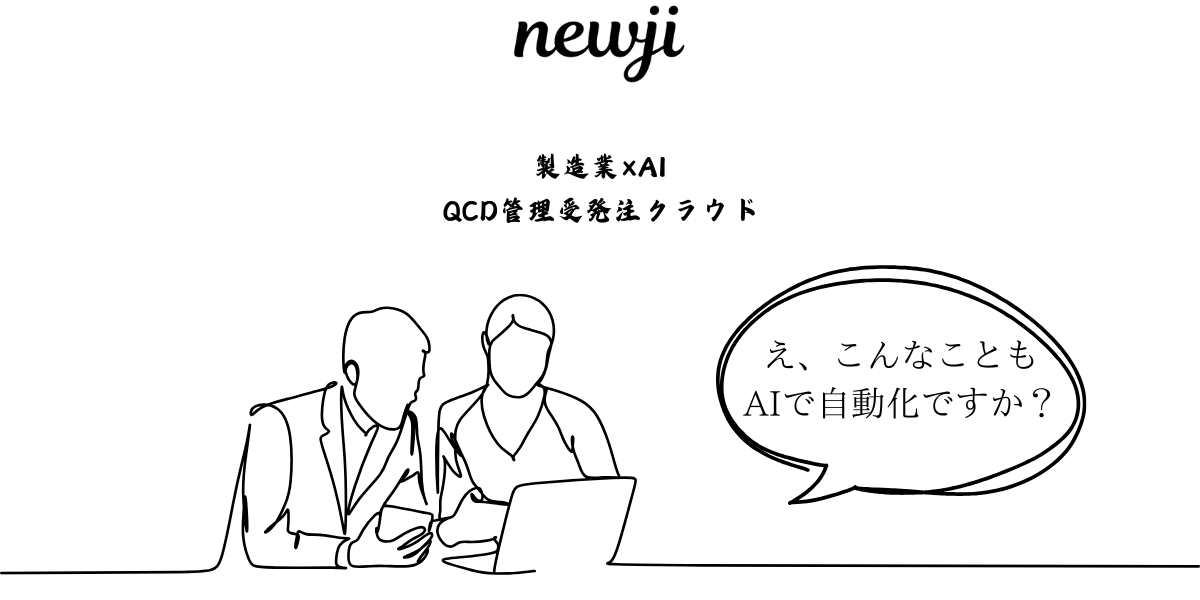
Markov chain Monte Carlo method (MCMC method)
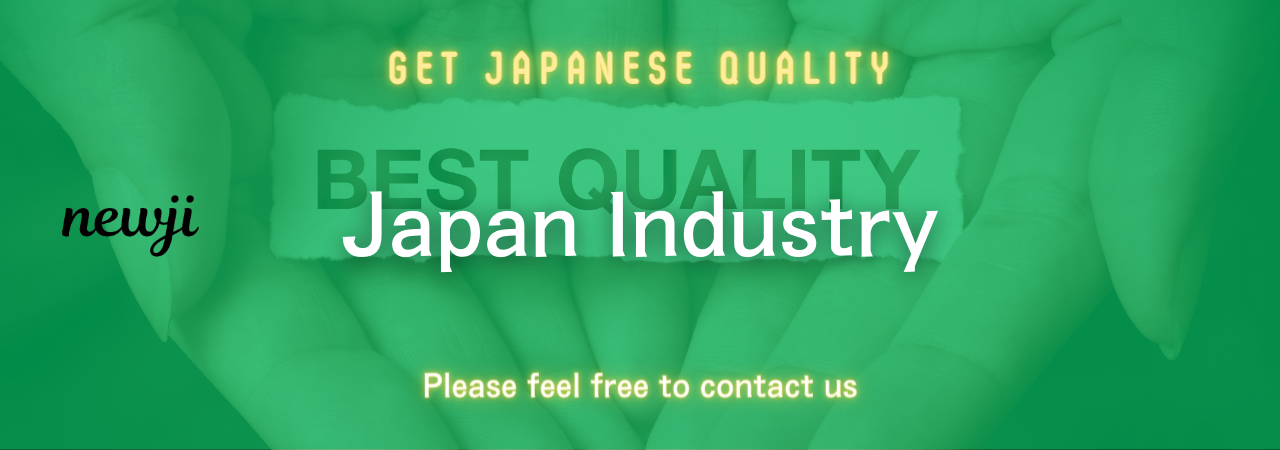
Understanding the Markov Chain Monte Carlo Method
The Markov Chain Monte Carlo (MCMC) method is an influential tool in the field of statistics and computer science.
It provides a way to approximate complex probability distributions and perform statistical inference in situations where traditional methods fall short.
Understanding its basic principles and applications can greatly enhance your grasp of data analysis and statistical modeling.
What is the MCMC Method?
The MCMC method is a class of algorithms that rely on constructing a Markov chain to obtain a sequence of random samples from a probability distribution.
This sequence converges over time to the desired distribution, allowing researchers to make inferences about the data.
The primary goal of MCMC is to approximate the distribution when direct sampling is not feasible, usually due to the high dimensionality or complexity of the model.
Components of MCMC
There are several key components of the MCMC method that form the backbone of the technique:
1. **Markov Chain**: A sequence of possible events where the probability of each event depends only on the state attained in the previous event. This memoryless property simplifies complex systems by reducing dependencies among events.
2. **Monte Carlo**: Refers to using randomness to solve problems that might be deterministic in principle. It’s a technique that relies on repeated random sampling to obtain numerical results.
3. **Stationary Distribution**: The target distribution we aim to sample from. The Markov chain is constructed such that it has this distribution as its equilibrium.
4. **Transition Kernel**: Defines how the process moves from one state to another and ensures that the stationary distribution is maintained.
How Does the MCMC Method Work?
The MCMC method involves generating samples that approximate the target distribution.
Here’s a basic outline of how it works:
1. **Initialization**: Start with an initial random state. This doesn’t need to be complicated or close to the target distribution.
2. **State Transition**: Using a proposal distribution, generate a candidate state from the current state. The proposal distribution might be symmetric as in Metropolis algorithms or might adjust as in Metropolis-Hastings algorithms.
3. **Acceptance Rule**: Determine whether to move from the current state to the candidate state. This is often decided using an acceptance probability, which ensures that over an infinite number of steps, the samples converge to the target distribution.
4. **Iteration**: Repeat the state transition and acceptance step. The longer the chain, the better the approximation to the target distribution.
5. **Convergence**: Eventually, the distribution of states in the chain becomes stable, thus approximating the target distribution.
Popular Algorithms in MCMC
There are several popular algorithms within the MCMC framework that are widely used:
1. **Metropolis Algorithm**: Proposed in 1953, it samples candidate states based on a symmetric proposal distribution. It uses an acceptance rule to decide whether the move to the candidate state should be made.
2. **Metropolis-Hastings Algorithm**: An extension of the Metropolis Algorithm, which generalizes the proposal distribution to be asymmetric. This is useful when the symmetry condition of Metropolis is not feasible.
3. **Gibbs Sampling**: A special case of the Metropolis-Hastings, it samples from the conditional distributions. It’s ideal when these conditional distributions are known and simplifies the sampling process.
4. **Hamiltonian Monte Carlo**: This method uses concepts from physics to guide the sampling process and is renowned for its efficiency in dealing with high-dimensional spaces.
Applications of the MCMC Method
The MCMC method is highly versatile and finds applications in various fields:
– **Bayesian Inference**: MCMC is crucial for Bayesian analysis, where it allows for the estimation of posterior distributions that are otherwise hard to compute analytically.
– **Machine Learning**: MCMC helps in estimating complex models, such as neural networks, by providing samples from the posterior distributions.
– **Statistical Physics**: It helps simulate systems at equilibrium, providing insights into the properties of materials and phenomena.
– **Genomics**: In genetics, MCMC is used to model genetic linkage and understand the genetic basis of diseases.
– **Econometrics**: Researchers use it to assess economic models and to forecast financial trends.
Advantages and Limitations
The MCMC method holds significant advantages:
– **Flexibility**: It can approximate any distribution regardless of its shape or dimensionality.
– **Scalability**: It’s effective even as the number of dimensions increases.
However, there are limitations:
– **Convergence Time**: It may require a large number of steps to reach convergence, impacting computational efficiency.
– **Chain Misbehavior**: Improper setup can lead to non-representative samples, impacting the validity of results.
Conclusion
The Markov Chain Monte Carlo method is a powerful tool for statistical analysis.
By understanding its components and how it works, you can apply it effectively in areas ranging from academics to industry.
While it presents some challenges, mastering MCMC can open the door to advanced statistical modeling and complex problem-solving.
Arming yourself with this knowledge prepares you for innovative applications and groundbreaking discoveries in the realm of data science.
資料ダウンロード
QCD調達購買管理クラウド「newji」は、調達購買部門で必要なQCD管理全てを備えた、現場特化型兼クラウド型の今世紀最高の購買管理システムとなります。
ユーザー登録
調達購買業務の効率化だけでなく、システムを導入することで、コスト削減や製品・資材のステータス可視化のほか、属人化していた購買情報の共有化による内部不正防止や統制にも役立ちます。
NEWJI DX
製造業に特化したデジタルトランスフォーメーション(DX)の実現を目指す請負開発型のコンサルティングサービスです。AI、iPaaS、および先端の技術を駆使して、製造プロセスの効率化、業務効率化、チームワーク強化、コスト削減、品質向上を実現します。このサービスは、製造業の課題を深く理解し、それに対する最適なデジタルソリューションを提供することで、企業が持続的な成長とイノベーションを達成できるようサポートします。
オンライン講座
製造業、主に購買・調達部門にお勤めの方々に向けた情報を配信しております。
新任の方やベテランの方、管理職を対象とした幅広いコンテンツをご用意しております。
お問い合わせ
コストダウンが利益に直結する術だと理解していても、なかなか前に進めることができない状況。そんな時は、newjiのコストダウン自動化機能で大きく利益貢献しよう!
(Β版非公開)