- お役立ち記事
- Predictive Maintenance! Reducing Downtime in Manufacturing!
Predictive Maintenance! Reducing Downtime in Manufacturing!
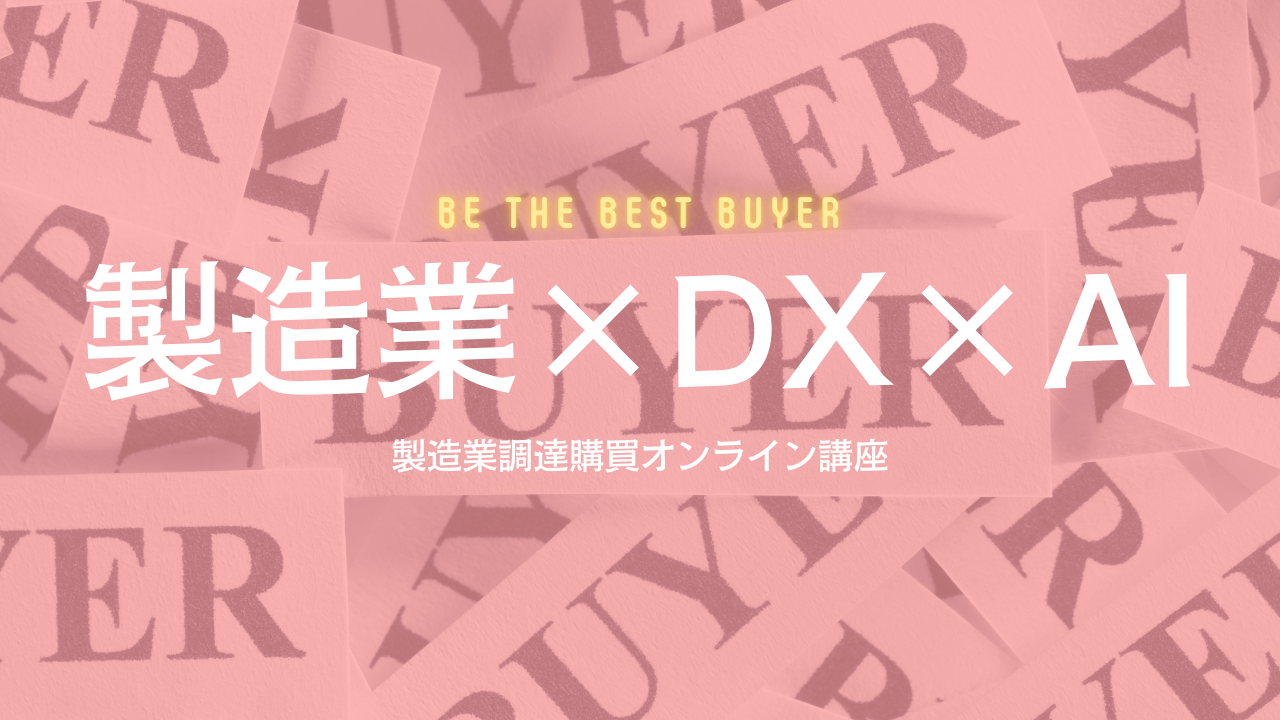
Predictive maintenance is a really helpful technique that companies can use to prevent their machines from breaking down. When machines break, it causes big problems. The company has to stop production until the machine is fixed. They lose money every minute it’s not working. Customers have to wait longer for their orders to be filled.
Predictive maintenance helps fix problems before they become big breakdowns. It uses sensors and data collection to monitor machines as they work. The sensors check things like vibration, temperature, oil quality and more. They send this information to computer programs that analyze the data for patterns. The patterns can predict when parts are starting to wear out or fail. Engineers can then change or repair those parts before a full breakdown occurs.
Let’s look at an example. imagine a company that makes bottle caps. They have a machine that pushes bottle caps onto bottles rolling by on a conveyor belt. One of the gears inside the machine is starting to wear out after months of continuous use. Through vibration sensors, the predictive maintenance program notices the gear is rattling more than normal. The data shows the rattling is getting louder each week. Engineers know, based on past machine data, that a loud rattling gear often means it will fail within the next month.
Rather than waiting until it breaks down fully, they schedule time to change the gear before the next weekend. Swapping it out takes just a few hours, and the machine is running again without issues. If they had waited for it to fully fail, it might have taken days to get the necessary replacement part and complete repairs. The production line would have been stopped the whole time, costing lots of money in lost bottles and overtime to catch up. Thanks to predictive data, downtime and costs were avoided!
The sensors provide continuous health monitoring of each machine. As small issues develop, early detection allows gradual, scheduled maintenance. This is much better than unexpected breakdowns. Waiting until visible failures occur often means more extensive, complex repair work too. Predictive maintenance reduces costs and increases productivity and profit. It also ensures predictable maintenance schedules rather than disruptive breakdowns. Employees can plan their work better as well.
Let’s discuss some common machine sensors:
– Vibration sensors: As described in the gear example, vibration analysis can detect imbalance, misalignment, loose components and more. Different vibration patterns indicate specific issues.
– Infrared sensors: Thermal cameras or contact probes monitor machine surface temperatures. Hot spots may mean increased friction from wear. Too much heat also shortens lifespan.
– Oil analysis: Samples check oil quality, coolant conditions, and look for metal fragments. This finds internal wear early before failures occur.
– Acoustic sensors: Microphones detect unusual sounds from fluid flow, bearing noise or other operations. Sounds indicate flow restrictions, component rubbing and more.
– Strain gauges: These flexing sensors detect forces on critical parts. Excess strain warns replacements may soon be needed.
– Ultrasound tools: Similar to medical ultrasound, these monitor thickness of critical metal components for signs of corrosion or erosion over time.
– Particle collection: Filters or oil samples check for microscopic metal fragments. Increased wear debris is an early warning sign.
– Pressure/flow sensors: Abnormal readings can mean valve issues, pump cavitation or other hydraulic/pneumatic problems developing.
– Electrical monitoring: Variable frequency drives, control systems and motors have sensors tracking parameters. Trending data finds control or efficiency issues.
The data from these various machine sensors streams digitally to the predictive maintenance software program. There, it is analyzed using techniques like machine learning, artificial intelligence, statistical analysis or other algorithms. Patterns can detect very subtle changes indicating needed repairs long before obvious failures occur. Over time, as more machine operational data is analyzed, the program gets better at predicting maintenance needs.
Predictive maintenance allows companies to plan service periods when it is most efficient rather than waiting for emergencies. Downtime is minimized. Spare parts are on hand when needed. The right technicians can be scheduled. Repairs are less complex so costs are lower too. Overall equipment effectiveness and productivity are greatly increased through this proactive approach. Manufacturing output becomes more consistent and reliable for customers as well. While the upfront investment may seem high, predictive maintenance saves huge amounts in avoided costs from unexpected downtime over time. It is a very smart strategy for any company relying on machinery to minimize risks to their operations. With robots and sensors, even home equipment may one day be monitored this way too!
資料ダウンロード
QCD調達購買管理クラウド「newji」は、調達購買部門で必要なQCD管理全てを備えた、現場特化型兼クラウド型の今世紀最高の購買管理システムとなります。
ユーザー登録
調達購買業務の効率化だけでなく、システムを導入することで、コスト削減や製品・資材のステータス可視化のほか、属人化していた購買情報の共有化による内部不正防止や統制にも役立ちます。
NEWJI DX
製造業に特化したデジタルトランスフォーメーション(DX)の実現を目指す請負開発型のコンサルティングサービスです。AI、iPaaS、および先端の技術を駆使して、製造プロセスの効率化、業務効率化、チームワーク強化、コスト削減、品質向上を実現します。このサービスは、製造業の課題を深く理解し、それに対する最適なデジタルソリューションを提供することで、企業が持続的な成長とイノベーションを達成できるようサポートします。
オンライン講座
製造業、主に購買・調達部門にお勤めの方々に向けた情報を配信しております。
新任の方やベテランの方、管理職を対象とした幅広いコンテンツをご用意しております。
お問い合わせ
コストダウンが利益に直結する術だと理解していても、なかなか前に進めることができない状況。そんな時は、newjiのコストダウン自動化機能で大きく利益貢献しよう!
(Β版非公開)